Machine Learning
The first aspect of advanced AI to be adopted in business was the concept of machine learning. Rather than gathering data and programming a computer as was done traditionally, machine learning allows the computer to gather its own data and, in a sense, program itself. By making complex connections between data, the computer can create new useful information.
A common misconception is that machine learning is simply about automation. That’s not really the case. We have had computer automation for decades, but only through extensive programming of each “expert system” scenario, use-case and situation that the automation would encounter.
Machine learning often begins with a basic decision tree that can be built out into a full neural network of connected data sets. Dealing with large amounts of data and acting on programmed rules are where machine learning out-paces the human mind.
A particularly effective application is seen in Facebook’s learning algorithms. By tracking a user’s clicks, searches, likes and time spent reading articles and posts, the algorithm creates a dynamic constantly updating picture of the person’s interests, hobbies, political views, travel plans and many other data points. This data then informs Facebook’s delivery of new content. The system then takes that user profile and curates custom content and advertising that is designed to appeal directly to the user.
Each user profile is then combined with the larger set of other user profiles to produce an ongoing constantly refined AI system that learns from user activity and responds with ever-improving suggestions for content or custom-tailored advertising.
Under less-advanced modeling, there would still be results, but they aren’t as refined and intuitive. For example, if a person clicks like on a post about Game of Thrones, it is simple for a computer program to lookup the metadata on Game of Thrones and determine that it is a TV show in the fantasy genre. This simple algorithm might then recommend Lord of the Rings.
But a more complex machine learning system can go much deeper. Facebook’s newest tracking algorithm tracks every interaction with the site and grades them according to the type of interaction and how “meaningful” they are. While that sounds like a nebulous concept, it’s really just a distillation of a large range of data, including likes, comments, clicks, sharing, time spent reading, and dozens of other factors.
Our example of Game of Thrones to Lord of the Rings might learn that people who like both of those things also are likely to like other unrelated things. Perhaps a substantial number people who are fans of these also tend to drive Honda Accords. The real systems obviously employ more variables than the three we are using for this example, but bridging the obvious connections with the not-so-obvious is the key to successful application of this type of algorithm.
Stumbling Blocks of Early Adopters
Learning about users and customers is an incredibly valuable and important part of business. But at this point, the early adopters are focusing on information aggregation and delivery. Acting intelligently on that information is the next step. Target found this out the hard way. Marketing personnel at the big box retailer knew that new parents are a gold mine of opportunity for their type of store. Their ability to cater to new parents can literally lead to a lifetime of customer loyalty from parent and child. Studies have also shown that this time in an adult’s life is when brand loyalty is most flexible. So Target’s machine learning was set to the task of determining when a customer became pregnant.
Purchases such as pregnancy tests and prenatal vitamins could easily be tied to future purchases. This means a customer who buys maternity clothes in July will probably be shopping for car seats in September, diapers in October, one-year old clothes a year later, and so on. This carries forward to school supplies five to six years later and eventually toys, electronics and everything else a family with children buys.
Using the first few indicators as a baseline, Target began offering ads to customers who appeared to be expecting. The results were good, but ran the company into a little trouble over privacy concerns when it mailed a pregnancy-related promotional ad to a teenage girl who had not yet told her father that she was pregnant.
The ability to capture a customer profile and tailor products directly is an important step in improving customer satisfaction and sales, but acting on that information correctly is something that can still benefit from the human touch. That’s one reason why AI and machine learning arw not so much autopilots as they are co-pilots that still requires extensive human guidance.
Analyzing the Real Costs and Savings
The rapidly improving ability of computers to analyze and act on new data is changing things everywhere in the business world. However, the real measure of a successful application of machine learning comes in the benefit per dollar spent.
While the eventual goal of deploying machine learning is that you can do the same job faster or better, the decision must be based on total cost, not just speed and quality of work. Imagine an AI program that can automate a job but actually takes 10 times longer to complete the work that human would. That sounds like a poor investment and a waste of resources.
But what if you factor in cost? If the machine learning program and all its maintenance and hardware costs amount to just one twentieth of the total cost of a human doing the job, then there is still a relevant use case that applies to many business problems. In this scenario, you can actually deploy 10 instances of the program and complete the same work for half the cost.
What Machine Learning Can’t Do (Yet)
Practical application of the principles of AI and machine learning are still in their infancy, but the possibilities seem endless.
There’s where some businesses run into problems. Machine learning programs can do things no human can do, like analyze years of data in a few seconds and display results and patterns. But humans are much better at determining if the results make sense in context. Humans also use intuition to respond to smaller data sets, which is something computers have no context for.
The solution to suboptimal machine learning framework is often to inject more and more quality data to help the system see more patterns. In one example, different teams competing to create a new predictive system for Netflix to recommend movies found that their models worked best when they were able to combine all the data sets that each team was using. This is useful for areas where you have a large dataset, but it slows the adoption of machine learning in a brand new field of study.
Optimized processes done by humans may not experience much improvement when an AI attempts to do the same job. The cost of “teaching” the system by gathering and inputting data and programming the rules for the data could be cost-prohibitive.
One famous recent example of machine learning in action was when IBM’s Watson machine learning program faced off against Jeopardy! champions Ken Jennings and Brad Rutter. Armed with an extensive set of encyclopedias and other text, Watson did very well against two of the game’s best human champions and defeated them in a two-game tournament in 2011. But from a pure business perspective, the multi-year efforts of a team of more than 15 data scientists was not a successful venture. Why? Because Watson’s winnings totaled just $1 million, which is far less than the cost of the project.
Obviously, in the Watson case money was not the goal. The $1 million went to charity and the win provided extensive marketing value to IBM while introducing the world to a new level of AI. But that type of project is not in the cards when making a case for real-world business AI.
The reality is that aside from a few mature applications such as monitoring large IT systems and data centers, email and spam filtering, travel time and traffic analysis by apps such as Waze or Uber, and other applications of large amounts of data, many AI and machine learning tools are still in the pilot-program stage.
At this part of the product lifecycle, they can do a few interesting things, but are not able to produce the total bang-for-the-buck that will revolutionize business. Other existing tools work in a limited fashion but still need extensive human interaction – known as supervised learning — to support and verify the results. These tools often produce only marginal cost savings. These products exist because they fill a niche for the companies that are looking to leverage the latest buzzword – “machine learning” in this case – before they have a complete AI-focused business enterprise strategy in place.
Natural Language Processing
Programming AI to understand the nuances of human speech and respond has been a goal since even before the original Star Trek showed Mr. Spock speaking to his workstation. Alan Turing, a pioneer in computer programming wrote an article in 1950 suggesting that the ability of a computer to process language and respond to conversation in such a way that it was indistinguishable from a person would be an important criterion in the development of artificial intelligence.
Early experiments with translation in the 1950s showed enough promise that some scientists thought they would crack the problem within a decade, but actual progress was much slower, and real application of practical natural language processing would come around the turn of the 21st century.
The ability of an AI to comprehend typical human speech patterns is known as Natural Language Processing. In the past decade, breakthroughs in processing speed, neural networking and deep machine learning have helped computer programs several quantum leaps forward. Using powerful rule-based statistical modeling, the AI can recognize words and develop a level of confidence in their meaning. Continued exposure to additional data points through processing of recorded speech or even live interaction with people allows the system to test and improve its results.
Effective natural language processing requires the ability to teach the system things and relationships that are used in human speech and programming in structures that people take for granted. Ascander Dost, a senior software engineer and linguist at Salesforce explains: “You know that a dog is a particular type of mammal, and a mammal is an animal, and there are other ones, and you know what hierarchy they form. Same with locations. A street occurs in a city, and a city and a town and a village are all the same thing, and they show up in a county, and the thing that contains the county is a state, and the thing that contains a state is a country. That’s all information that we carry around in our heads about the world based on our interactions with it.”
That type of ontological information gives the system a framework to deal more successfully with language concepts and some abstract ideas, moving from a straight natural language processing function towards real natural language understanding.
What Natural Language Processing Does for Consumers
Early applications for consumer use were in call center triage, where standard interactions moved from “press 0 for Operator” to “say Operator.” But this type of language processing also has applications in predictive text that assists in search engines. Google’s search algorithms can predict the most likely results not just from a list of keywords, but from a full natural sentence. Google’s engine understands both “restaurant Times Square Italian” and “Where can I eat pasta in Times Square?”
This processing also occurs in predictive text and autocorrect. By comparing common sentences, these functions are able to successfully guess what your next word will be or what you meant to type. The results aren’t always great, but these systems continue to get better over time.
One of the most interesting horizons in natural language processing is the use-case for personalized service based on your own data. One example would be a health insurance company, which already has a substantial log of data about you. When you can pull from that large dataset and provide meaningful recommendations, you can provide an automated concierge service.
Examples of this in action might be typing (or verbalizing) a question like: “I need to see a doctor in my plan that treats sports injuries.” With the data at hand, the language processor can search for a doctor that fits that criteria. Or, it might have to ask additional questions, such as: “I have listings for doctors who specialize in sports medicine. Let’s narrow your symptoms down. What type of injury are you treating?” When the person responds that they have knee pain or a shoulder injury, the system would search for doctors with more experience treating that type of injury.
These are the types of questions that a human attendant would have a great deal of success handling, but their search might take longer and they might need extensive training to deal with customers. A phone operator is also a single channel whereas an AI program can serve multiple customers simultaneously.
Natural Language Processing in Business
Over the past few years, many of us have gotten used to applications of AI that talk to us. Natural Language Processing makes that possible both through speech and text. Personal assistant applications like Siri, Google Home and Alexa can answer questions, automate the home and look up information for us. But these tools are rudimentary and limited. Compared to what is coming, they are merely a step above a proof-of-concept. These functions can be applied to large datasets of any kind, allowing users and customers to get answers to increasingly complicated questions.
Many large companies have realized that they capture and store a great deal of unstructured text data. In comparison to numerical data, text data must be parsed by a natural language processor in order to use it effectively. This ability is improving every day and opens up the possibility of affecting business in a variety of ways. One mundane application might be processing email messages and sorting those that ask a question, then prioritizing or reminding the recipient to respond to the question. A more advanced application might be in the creation of automated data retrieval functions that access large sets of information based on an open-ended question. Rather than programming a complex database query, you could say “what are revenue trends for the past three quarters?” Your virtual data scientist would then compile and display that data. Forming that question in words is much easier for most people that typing formulas or complex programming.
One emerging application is the use of customer-service “chatbots” to help triage and organize customer interactions. By providing a computer program on a website that can respond to customer concerns and questions, businesses can provide more effective and proactive customer support. These AI programs can respond to natural-language inquires such as “How do I return an order?” with human-like responses. They are also able to escalate more complex problems to traditional customer service reps, providing a seamless blend between human and AI representatives.
Natural Language Processing is also helping to advance the field of translation. Computer-aided translation technology has struggled for decades to produce results that as good as a person who understands the nuances of a native speaker. The ability to do this is now close at hand. Results can still occasionally be a bit awkward, but they are improving at a rapid pace.
Another example of a useful business application is the monitoring, compilation and extraction of information from social media channels. By processing what is written out on the internet, a natural language program can generate conclusions about company reputation, marketing successes and failures and can create a general picture of how your business is perceived. This information can also by paired with demographics, market research and competitor research to create a big picture of your product or service’s place in the market. This helps with ad placement and media engagement.
With the right kind of data, this process can even analyze the sentiments and emotions expressed by people who interact with your company. As with most machine learning and AI applications, this is taking a very large task and automating much of data collection and processing to allow the expert personnel in that field to draw conclusions based on much larger compilations of data.
When Natural Language Processing Doesn’t Work
Consumer acceptance of talking computers and natural language processing is increasing all the time. But when it doesn’t work perfectly, people tend to lose patience very quickly. Watch someone with an iPhone talk to Siri and you might see them quickly grow impatient.
Experts say voice assistants tend to have about a 95% success rate – which sounds good – but the one in 20 words it misses are enough to turn users off to the whole experience.
One solution to improve the comprehension rate is to employ machine learning and help the language processor improve itself as it goes. But there can be downsides to that, as Microsoft found when it let its Tay chatbot absorb Twitter content. Within a day, Tay was tweeting offensive remarks it generated based on its unfiltered input from Twitter users.
Natural Language Processing also falls short when considering the wider range of language beyond the developed world. Each of the world’s languages is a new problem starting from the ground up. Businesses hoping to deploy chatbots in many developed countries will find a lot of capability in processing the dominant language in those areas, but in the developing world – which is beginning to serve more and more internet customer every day – natural language processing is lagging.
Computer Vision
Computer analysis of a visual image has achieved incredible improvements in the past decade. The ability to analyze a digital image and draw conclusions from it is supported by AI, neural networks and machine learning.
When a human sees an image, the way we interpret it is informed by our experience and memories. To us, this happens automatically, but for a computer to get the same information, it needs to be provided to it somehow. But AI is now helping computers to not only store and retrieve the “experience” of looking at images, but also allows it to interpret and intuit the content of new images based on that stored information.
This type of supervised learning using digital imagery has actually progressed leaps and bounds beyond other areas of AI development. At the beginning of the century, a task as simple as recognizing objects, people or animals and counting them in an image would have been beyond most image recognition software.
This revolution has started to change more than one industry and it’s only just beginning to affect the way we live and do business.
Security
Active monitoring of surveillance camera feeds is a manual labor intensive process. Conducting forensics based on camera footage after the fact is also a difficult process for law enforcement. But advances in computer vision provide useful applications for facial recognition and automated alerting based on certain types of movement and behavior.
Recent and ongoing advancements allow computers to analyze live video and categorize events while escalating appropriately after detecting specific actions. Potential future application for law enforcement might provide the ability to load a mugshot into its system and virtually tail a person around town.
Casinos are already deploying facial recognition to catch people who have been banned for attempting to cheat and motion analysis to catch people in the act. This technology will improve in both its skill and scope as AI improves.
Current consumer-grade and small enterprise “smart” cameras can alert on motion, but produce a lot of false positives. They can let you know when a break-in is about to occur but they also alert on birds or trees blowing around in the wind. The next generation of surveillance available in the home or small business will be able to recognize specific people, objects, vehicles and even behavior.
Agriculture
In the United States, AI is already starting to revolutionize agriculture. At least 10% of certain crops are already being grown under the supervision of machines. The ability to capture an image and compare it to known conditions has already begun to improve yields and cut costs in certain areas. More data gathered and the ability to efficiently process it helps guide farming in real time.
Drones outfitted with sophisticated cameras can fly above a field and take pictures over time. Comparing the imagery allows for early detection of pests, weeds and helps to measure growth. Over time, this will help improve production models and planting strategies by tying in directly to yield-boosting AI algorithms.
Manual cultivation of lettuce requires careful thinning of seedlings just before fertilizing the fields. A new system has been developed to handle this labor-intensive work. The machine, which is essentially a tractor fitted with an imaging system, is able to drive over the small lettuce plants and use its AI to analyze the images it takes. The AI is able to determine which plants should be fertilized, which lettuce plants should be thinned and if any weeds need to be killed. The results have met or exceeded the standard set by traditional methods of sending workers into the field to examine each plant.
Other systems are available to automatically harvest strawberries and apples at just the right time. They rely on the imaging systems to determine where the fruit is and how and when to pick it.
These tools are now providing more and better information to farmers while also saving money, time and manual labor.
Medicine
Much of the R&D directed towards AI comes in the medical community. As a result, some of the first early successes are happening in that field.
In Germany, a team has tested a machine that can predict skin cancer by examining photographs of a patient’s mole or other skin blemish. Using supervised machine learning, the system was exposed to a large number of photographs of actual cancerous lesions that had been identified by expert doctors and then tested.
After this learning phase, the system then examined as series of new images that it had not seen and asked to recommend which blemishes should be examined more closely and sent for a biopsy. A group of expert physicians were also asked to score the same photos.
After reviewing thousands of photographs, the system learned to detect cancer at a 95% success rate – compared to 86% for a team of doctors reviewing the same photographs.
“(The machine) missed fewer melanomas, meaning it had a higher sensitivity than the dermatologists (and) misdiagnosed fewer benign moles as malignant melanoma … this would result in less unnecessary surgery.” one of the study’s authors said.
Overall, the human dermatologists’ success rate improved when they were permitted to review the patient histories and other information, showing that the computer vision system may function well as an early detection system that can refer patients for further examination or treatment.
Extending these functions to reading 3-D CT scan and MRI imagery can also help diagnose and study a large range of medical issues.
Transportation
Transportation of goods and people has slowly innovated by adopting advanced technology. The major challenges of maintaining safety and reliability have slowed some adoption of mature technologies.
The logistics field has long made use of machine-readable codes such as barcodes or RFID chips. It also has already adopted sophisticated internet-of-things technology that helps track and monitor vehicles or shipments at every step of the logistics chain. By integrating computer vision applications with existing tracking flow systems, shipping and receiving functions can improve accuracy, loading times and inventory analysis. The newest computer vision applications can actually recognize objects or measure amounts just through the use of cameras and AI. This assists in verification of shipments and delivery throughout the entire supply chain and can also be used for accurately taking inventory.
In transportation, the most well-known development has been in the testing of self-driving cars. Complete application and adoption of self-driving vehicles is a few years away. But computer vision is already on the road, aiding in lane-keeping collision avoidance and self-parking. Additionally, warehouses are already deploying autonomous or nearly autonomous forklifts and other machines that can find and organize items more quickly than humans. Delivery services that use autonomous drones have also been promised.
All of this is made possible by the machine’s ability to see and interpret digital images as well or better than a human. Like other applications of AI, computer vision is also in its infancy. But over the next few years it will continue to improve and provide more and more opportunity to incorporate it in business functions.
Big Data Investment
The most apparent use case for AI and machine learning is in the fields of Big Data. A traditional business analytics investment can return as much as $13 per dollar spent. Analysis and processing of large sets of data only becomes easier and more feasible and practical when machine learning contributes to the intelligent analysis and parsing of disparate databases.
However, properly devising a big data strategy can be a complicated and expensive undertaking. Each problem is unique and each solution takes careful thought and design before you can even begin.
Early adopters tend to be larger companies that have the capital to risk and experiment and plenty of experience in the tech sector. Among those that have taken these early steps, about 22% have high revenue growth and profitable outcomes, while 31% have not witnessed a measurable return on that investment yet.
What’s Next?
As machine learning, natural language and computer vision services become more mainstream, they will continue to automate existing processes, provide greater insight and bring big data to big problems.
Early adopters have mixed feelings on the abilities of AI tools, but businesses that are beginning to leverage AI now will have a leg up when truly revolutionary developments come online in the near future.
People who have a good knowledge of AI expect it to be as commonplace as electricity. As Stanford professor Andrew Ng said earlier this year: “About a century ago, we started to electrify the world through the electrical revolution. By replacing steam powered machines with those using electricity, we transformed transportation, manufacturing, agriculture, healthcare and so on. Now, AI is poised to start an equally large transformation on many industries. … The only industry which will not be transformed will probably be hairdressing.”
How to Get Started
With all the changes that are coming, there is a fine line between the expense of becoming an early adopter and the cost of falling behind because you waited too long.
Machine Learning and AI are helping to hard-code human expertise and knowledge into rule-based and evolving systems. Adopting an AI system because it’s nice to have a new toy or to run a pilot project doesn’t usually make sense for most businesses.
Businesses should approach the adoption of AI technology that is already mature and that is able to automate an ongoing business function.
Areas that make sense for early adopters include:
Network Monitoring: Automation of alerting systems is easily managed. A properly configured AI system monitor can cut down on the routine work of systems administrators and free up time and resources to focus on major issues.
Cybersecurity: Going hand-in-hand with system monitoring is the application and updating of a network’s security posture. An AI can monitor every endpoint on your system at the same time and report on possible hacking attempts or needed updates to operating systems or software.
Customer Service: Triaging the minor problems and helping to categorize and deal with day-to-day customer interactions can go a long way towards creating happy customers and freeing up trained staff to deal with more important issues.
Logistics: There are multiple way for a supply chain to take advantage of automation, tracking and data analysis to improve all levels of service.
Healthcare: While still in its early stages for diagnosis and patient monitoring, many in the medical community are employing AI and machine learning for image processing and data collection.
Finance: : Rules-based investment and monitoring is already operating in the banking and finance industry. AI and machine learning can also be employed for monitoring and detecting fraud activity, while computer vision is already in place to read checks and deposit slips at the automated teller machines and mobile apps of many banks.
The resources and personnel required to get a machine learning AI up and running are significant. Qualified data scientists and experienced machine learning designers are in demand and there is still a shortage of people in these fields.
But for many industries, now might be the time to take the first step forward and bring AI and machine learning into your business. The biggest question on the minds of anyone considering an artificial intelligence solution is how to measure and realize a return on that initial investment. We took a look at some of the successes seen by some of the early adopters and have created a report on Return on Investment in AI. Click the link to receive a copy of the report and contact us if you have any questions.
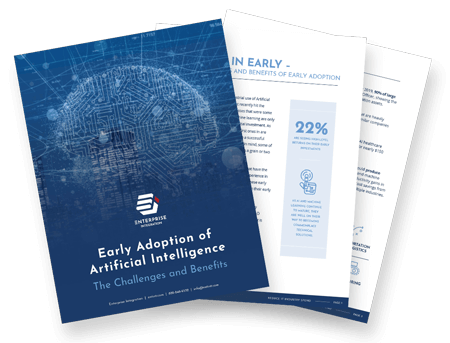
The next step is learning how to integrate AI and machine learning into your technological ecosystem. Doing so efficiently and effectively using proven models and methodology can open an entire world of automation and increased productivity. Many businesses also see a profound reduction in costs over the life of an AI or machine learning project. The key is careful planning, research and connections with the right kind of expert partners